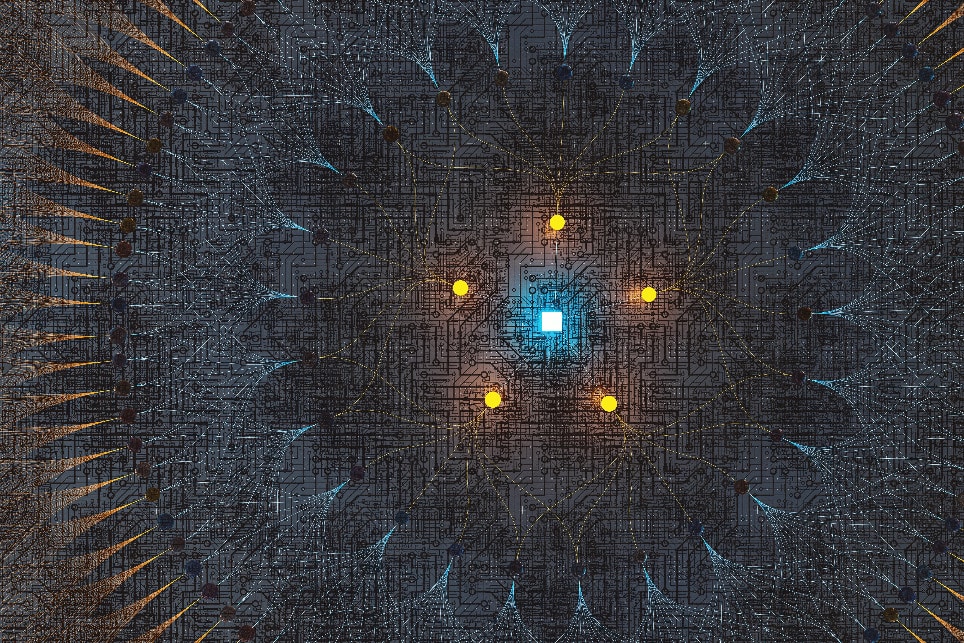
Quantum physicist Mario Krenn remembers sitting in a coffee shop in Vienna in early 2016, poring over printed pages from his computer, trying to understand what MELVIN had found. MELVIN was a machine learning algorithm that Krenn had built, a kind of artificial intelligence. His job was to mix and match the building blocks of standard quantum experiments and find solutions to new problems. And he found many more interesting ones. But there was one that didn’t make sense.
“The first thing I thought was, ‘My program has a bug, because the solution cannot exist,'” Krenn said. MELVIN apparently solved the problem of creating highly complex entangled states involving multiple photons (entangled states being those that once caused Albert Einstein to invoke the spectrum of “spooky action at a distance”). Krenn and his colleagues didn’t explicitly provide MELVIN with the rules it needed to generate such complex states, but they found a way to do it. Eventually, he realized that the algorithm had rediscovered a kind of experimental setup that had been created in the early 1990s. But these experiments were much simpler. MELVIN had cracked a far more complex puzzle.
We are a platform dedicated to knowledge that can only continue to exist thanks to its community of supporters. Find out how to help.
“Once we understood what was happening, we were able to immediately generalize [the solution],” said Krenn, who now works for the University of Toronto. Since then, other teams have begun to carry out the experiments identified by MELVIN, allowing them to test the conceptual underpinnings of quantum mechanics in new ways. Meanwhile, Krenn, Anton Zeilinger of the University of Vienna and their colleagues refined their machine learning algorithms. Its latest effort, an AI called THESEUS, promises to go further: it’s orders of magnitude faster than MELVIN, and humans can easily analyze its output. Although Krenn and his colleagues take days or even weeks to understand MELVIN’s antics, they can find out almost immediately what THESEUS is saying.
“It’s incredible work,” said theoretical quantum physicist Renato Renner of the Institute for Theoretical Physics at the Swiss Federal Institute of Technology Zurich, who reviewed a 2020 study on THESEUS by Krenn and Zeilinger but was not directly involved in those efforts. .
Krenn stumbled upon this entire research program somewhat by accident when he and his colleagues were trying to figure out how to experimentally create quantum states of entangled photons in a very particular way: when two photons interact, they become entangled, and both can only be mathematically described using a single shared quantum state. If you measure the state of one photon, the measurement instantly determines the state of the other, even if the two are miles apart (hence Einstein’s ironic comments about entanglement being “creepy”).
In 1989, three physicists – Daniel Greenberger, the late Michael Horne and Zeilinger – described an entangled state that came to be known as “GHZ” (after their initials). It involved four photons, each of which could be in a quantum superposition of, say, two states, 0 and 1 (a quantum state called a qubit). In their paper, the GHZ state involved the entanglement of four qubits so that the entire system was in a two-dimensional quantum superposition of states 0000 and 1111. If you measured one of the photons and found it in state 0, the superposition would collapse and the other photons would also be in state 0. The same applies to state 1. In the late 1990s, Zeilinger and his colleagues experimentally observed GHZ states using three qubits for the first time.
Krenn and his colleagues were looking for higher dimensional GHZ states. They wanted to work with three photons, where each photon has a dimensionality of three, which means it could be in a superposition of three states: 0, 1, and 2. This quantum state is called a qutrit. The entanglement the team sought was a three-dimensional GHZ state that was a superposition of states 000, 111, and 222. Such states are important ingredients for secure quantum communications and faster quantum computing. In late 2013, researchers spent weeks designing experiments on blackboards and doing calculations to see if their configurations could generate the necessary quantum states. But each time they failed. “I thought, ‘This is absolutely insane. Why can’t we create a configuration?'” Krenn said.
To speed up the process, Krenn first wrote a computer program that did an experimental setup and calculated the result. Then he updated the program to allow qLet him incorporate into his calculations the same building blocks that experimenters use to create and manipulate photons on an optical table: lasers, non-linear crystals, beam splitters, phase shifters, holograms, and the like. The program searched a large space of configurations by randomly mixing and matching the building blocks, performed the calculations, and displayed the results. And so, MELVIN was born. “In just a few hours, the program found a solution that we scientists – three experimentalists and one theorist – couldn’t find for months,” Krenn said. “It was a crazy day. I couldn’t believe this happened.”
So he gave MELVIN more intelligence. Whenever it found a setting that did something useful, MELVIN added that setting to its toolbox. “The algorithm remembers that and tries to reuse it for more complex solutions,” Krenn said.
It was this more evolved MELVIN that left Krenn scratching his head in a Vienna coffee shop. He set it up to work with an experimental toolbox that contained two crystals, each capable of generating a pair of entangled photons in three dimensions. Krenn’s naive expectation was that MELVIN would find configurations that combine these photon pairs to create entangled states of at most nine dimensions. But “he really found a solution, an extremely rare case, which has a much greater entanglement than the rest of the states,” Krenn said.
Eventually, he discovered that MELVIN had used a technique that several teams developed nearly three decades ago. In 1991, a method was developed by Xin Yu Zou, Li Jun Wang and Leonard Mandel, all from the University of Rochester, in the United States. And in 1994 Zeilinger, then at the University of Innsbruck in Austria, and his colleagues created another. Conceptually, these experiments attempted something similar, but the setup that Zeilinger and his colleagues developed is simpler to understand. It starts with a crystal that generates a pair of photons (A and B). The trajectories of these photons pass through another crystal, which can also generate two photons (C and D). The trajectories of photon A from the first crystal and photon C from the second exactly overlap and lead to the same detector. If this detector clicks, it is impossible to tell whether the photon originated from the first or second crystal. The same goes for photons B and D.
A phase shifter is a device that effectively increases the path a photon travels as a fraction of its wavelength. If you were to introduce a phase shifter into one of the paths between the crystals and keep changing the amount of phase shift, it could cause constructive and destructive interference in the detectors. For example, each of the crystals can generate, say, 1000 photon pairs per second. With constructive interference, the detectors would register 4,000 photon pairs per second. And with destructive interference, they would detect none: the system as a whole would not create any photons, even though the individual crystals would generate 1,000 pairs per second. “That’s actually pretty crazy, when you think about it,” Krenn said.
MELVIN’s amazing solution involved these overlapping trajectories. What confused Krenn was that the algorithm only had two crystals in its toolbox. And instead of using these crystals at the beginning of the experimental setup, he trapped them inside an interferometer (a device that splits the path of, say, a photon into two and then recombines them). After much effort, he realized that the configuration MELVIN had found was equivalent to one involving more than two crystals, each generating pairs of photons so that their trajectories to the detectors overlapped. The configuration can be used to generate high-dimensional entangled states.
Quantum physicist Nora Tischler, who was a Ph.D. working with Zeilinger on an unrelated topic at the time MELVIN was being tested, I was paying attention to these developments. “It was pretty clear from the start that such an experiment wouldn’t exist if it hadn’t been discovered by an algorithm,” she said.
In addition to generating complex entangled states, the configuration using more than two crystals with overlapping trajectories can be employed to perform a generalized form of Zeilinger’s 1994 quantum interference experiments with two crystals. Aephraim Steinberg, an experimentalist at the University of Toronto who is a colleague of Krenn’s but has not worked on these projects, is impressed by what AI has discovered. “This is a generalization that (as far as I know) no human being dreamed in the ensuing decades – and perhaps never – of conceiving,” he said. “It’s a wonderful first example of the kind of new explorations these thinking machines can take us.”
In a generalized configuration withWith three crystals, each generating a pair of photons and overlapping trajectories leading to four detectors, quantum interference can create situations where all four detectors “click” (constructive interference) or none of them “click” (destructive interference).
But until recently, carrying out such an experiment was a distant dream. Then, in a pre-published March paper, a team led by Lan-Tian Feng of the University of Science and Technology of China, in collaboration with Krenn, reported that they had fabricated the entire setup on a single photonic chip and performed the experiment. The researchers collected data for more than 16 hours: a feat made possible by the incredible optical stability of the photonic chip, something that would be impossible to achieve in a large-scale desktop experiment. To begin with, the setup would require a square meter of optical elements precisely aligned on an optical table, Steinberg said. Furthermore, “a single optical element oscillating by one-thousandth of the diameter of a human hair during those 16 hours may be enough to eliminate the effect,” he said.
During their first attempts to simplify and generalize what MELVIN had found, Krenn and his colleagues realized that the solution resembled abstract mathematical shapes called graphs, which contain vertices and edges and are used to represent pairwise relationships between objects. For these quantum experiments, each path a photon travels is represented by a vertex. And a crystal, for example, is represented by an edge connecting two vertices. MELVIN first produced such a graph and then performed a mathematical operation on it. The operation, called “perfect match”, involves generating an equivalent graph in which each vertex is connected to only one edge. This process makes calculating the final quantum state much easier, although it is still difficult for humans to understand.
This changed with MELVIN’s successor, THESEUS, which generates much simpler graphs by separating the first complex graph representing a solution that it finds down to the minimum number of edges and vertices (so any further exclusion destroys the configuration’s ability to generate the desired quantum states). These graphs are simpler than MELVIN’s perfect match graphs, so it’s even easier to understand any AI-generated solution.
Renner is particularly impressed by the human interpretable results of THESEUS. “The solution was designed in such a way that the number of connections in the graph is minimized,” he said. “And this is, of course, a solution that we can understand better than if you had a very complex graph.”
Eric Cavalcanti of Griffith University in Australia is impressed with the work and wary of it. “These machine learning techniques represent an interesting development. To a human scientist looking at the data and interpreting it, some of the solutions might seem like new ‘creative’ solutions. But at this stage these algorithms are still far from a level where you can say they are coming up with really new ideas or coming up with new concepts,” he said. “On the other hand, I think one day they will get there. So these are baby steps – but we have to start somewhere.”
Steinberg agrees. “For now, they are amazing tools,” he said. “And like all the best tools, they already allow us to do some things we probably wouldn’t have done without them.”